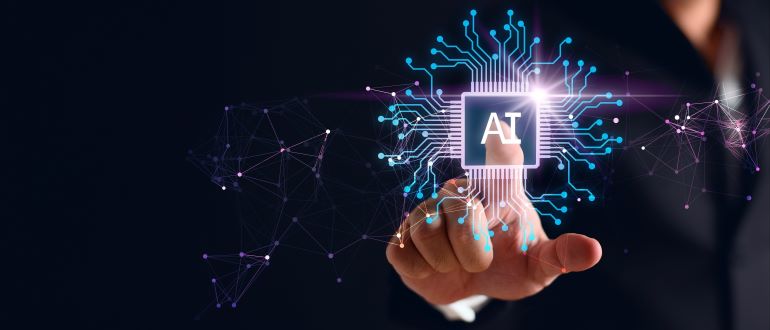
The Evolution of AI in Platform Engineering
Platform engineering has emerged as a critical approach for modern development teams, with Gartner predicting that 80% of software engineering organizations will establish dedicated platform engineering teams by 2026. As businesses increasingly adopt cloud technologies, AI in platform engineering has become crucial in handling complexities such as system integrations, performance optimization and technology adaptation.
The integration of AI is fundamentally transforming platform engineering by automating routine tasks and optimizing complex workflows. By 2025, AI will be deeply embedded into the development lifecycle, improving how platforms are designed, built and maintained while enhancing scalability, performance and intelligence.
Intelligent Automation: Beyond Basic Processes
AI takes automation to the next level by providing more innovative decision-making capabilities. With machine learning algorithms, platforms can optimize resource allocation, error detection and troubleshooting with minimal human intervention. These AI-driven tools analyze massive amounts of data to predict potential bottlenecks and automatically adjust system configurations for optimal performance.
This intelligence extends to Infrastructure as Code (IaC), CI/CD pipelines and self-healing systems that can automatically detect and fix failures, ensuring minimal downtime and improving platform reliability.
Code Generation: Accelerating Development
In 2025, AI tools will increasingly assist developers by generating code snippets and entire modules based on natural language descriptions. This significantly reduces development time and lowers the barrier to entry for developers, making it easier for teams to build complex systems without having to write every line of code from scratch.
According to industry research, developers who use AI code tools report a 20% to 50% increase in speed for everyday coding tasks. When tasks like boilerplate code writing, syntax corrections, or API usage suggestions are automated, engineers can focus on logic design, architecture and creative problem-solving.
Source: DevOps and Application Development 1H 2025 Decision-Maker Survey, January 2025
Predictive Analytics for Platform Health
AI is being used to monitor platform health in real-time, predict system failures and recommend solutions based on historical data and usage patterns. This proactive approach enhances platform reliability and reduces downtime by allowing platform engineers to address issues before they escalate.
For platform teams, this capability transforms their approach from reactive troubleshooting to proactive maintenance, ultimately delivering more stable and reliable systems to end users.
“AI-powered work is quickly demonstrating how AI can augment and perform a multitude of tasks across the software development lifecycle,” said Mitch Ashley, VP and practice lead, DevOps and application development at Futurum. “Platform Engineering, ITOps and System Reliability Engineering are proving to be primary candidates for AI-driven use cases.”
Enhancing Developer Experience
One of the primary purposes of platform engineering is to improve the developer experience.
An Internal Developer Platform (IDP) powered by AI can provide developers with pair programming tools, speeding up their work and enhancing code quality.
AI can also free developers from less valuable activities through no-code interfaces, allowing for more democratized and accelerated management of the entire software development lifecycle.
Addressing AI Integration Challenges
Despite its benefits, integrating AI into platform engineering presents several significant challenges:
Ethical Concerns and Bias
As AI capabilities evolve, ethical concerns regarding algorithm bias, fairness and potential misuse arise. If the input data is biased, the output will likely be biased as well. Development teams can prioritize fairness by ensuring that data sets used for training AI models are diverse and unbiased.
Data Privacy and Security
As organizations process increasing amounts of data, concerns arise that AI could fuel security breaches and expose private information.
Integrating AI into platforms can introduce new security vulnerabilities that malicious actors might exploit. Robust security measures, such as penetration testing and vulnerability assessments, have become critical in mitigating these potential risks.
Balancing Automation With Human Oversight
As AI systems become more sophisticated and integrated into various platforms, ensuring a harmonious relationship between automation and human involvement is crucial.
The maturity and trustworthiness of this technology can lead to resistance among adopters and heighten user concerns. Developers and platform engineers must determine the most effective way to work with intelligent systems to achieve optimal results.
Looking Ahead
The future of AI in platform engineering is promising, with technologies such as large language models (LLMs) continuing to evolve and integrate seamlessly with development workflows. The generative AI market is projected to grow from $11.3 billion in 2023 to $51.8 billion by 2028 at a compound annual growth rate of 35.6%, according to reports from Research and Markets.
As these technologies mature, we’ll see closer integration between AI tools and all aspects of the platform engineering lifecycle, from initial development to ongoing maintenance and optimization.