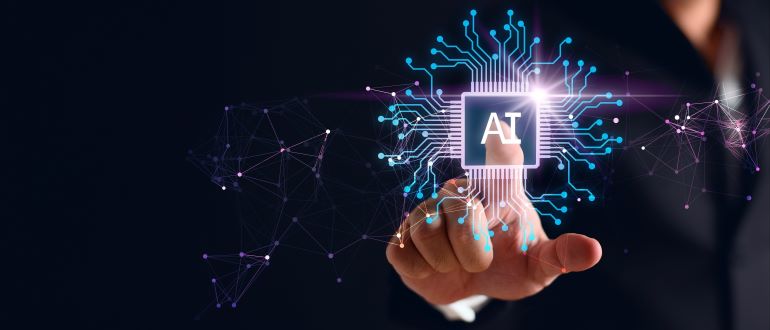
As artificial intelligence (AI) becomes increasingly integrated into enterprise operations, the demand for responsible, scalable AI practices is more pressing than ever. AI’s expansion – from predictive models to advanced generative capabilities – drives digital transformation, but it also introduces significant challenges, including data security concerns, model drift, bias and regulatory compliance.
In response, enterprises are turning to platform engineering to build AI systems that are not only efficient and innovative but also transparent, ethical and reliable.
The Need for Responsible AI
The rapid evolution of AI has led to unprecedented opportunities for growth and efficiency. However, it also raises critical ethical and operational questions. AI models are only as good as the data they are trained on, which makes data provenance and integrity vital. Issues like model drift – where AI performance degrades over time due to changing data patterns – pose additional risks. Furthermore, the lack of transparency in decision-making processes can lead to biased outcomes, potentially damaging brand reputation and customer trust.
Simultaneously, regulatory landscapes are shifting. Governments worldwide are introducing legislation aimed at mitigating the risks associated with AI. The EU AI Act, for instance, sets stringent requirements for transparency, accountability and data governance. Enterprises need to proactively address compliance challenges in this environment while maintaining agility and competitiveness.
Platform Engineering: The Foundation for Responsible AI
Traditional IT infrastructures or generic cloud solutions are ill-equipped to handle the complexities of modern AI operations. Platform engineering provides a solution by offering a comprehensive, scalable infrastructure tailored to AI development, deployment and monitoring needs.
This approach integrates observability and data governance directly into the AI/ML lifecycle, ensuring transparency, accountability and compliance at every stage.
Observability in AI Operations
Observability is crucial for maintaining control and performance throughout the AI model lifecycle. Advanced observability solutions provide:
- Comprehensive monitoring: By integrating metrics, events, traces and logs, observability tools offer a real-time, holistic view of AI model performance, helping detect anomalies and assess system behavior.
- Anomaly detection and pattern recognition: Leveraging generative AI models, observability solutions can identify deviations from normal behavior and proactively address issues before they escalate.
- Explainability and root cause analysis: These tools not only pinpoint deviations but also explain why they occurred, aiding in diagnosing problems related to model drift, data quality, or operational anomalies.
- Scalable infrastructure: Designed to grow with enterprise needs, observability solutions support an increasing number of models and complex data streams while maintaining performance.
- Collaboration and feedback loops: By centralizing observability data, these platforms enhance collaboration among data scientists, ML engineers, and DevOps teams, fostering continuous improvement and strategic decision-making.
Integrating Observability Into the AI Lifecycle
Effective observability spans the entire AI/ML lifecycle, ensuring consistent performance and compliance from development to deployment. Key phases include:
- Model training and development: Observability tools monitor data quality, algorithm performance, and hyperparameter adjustments, ensuring model integrity from the start.
- Model deployment: During the transition to production, observability tracks performance in real-world scenarios, enabling early detection of model drift or performance degradation.
- Continuous model monitoring: Post-deployment, continuous monitoring ensures models remain accurate and effective as they encounter new data or changing conditions.
- Feedback loops and collaboration: Observability promotes ongoing stakeholder collaboration, ensuring models evolve alongside business objectives and regulatory requirements.
To implement effective observability, enterprises should:
- Utilize a centralized observability framework to maintain visibility across all models and systems.
- Leverage cloud-native services for scalability and flexibility.
- Deploy observability instrumentation to automate data collection on performance and interactions.
- Establish monitoring and alerting systems to respond to anomalies or deviations rapidly.
- Invest in explainability and root cause analysis tools to maintain transparency and accountability.
Proactively Addressing AI Legislation
The EU AI Act exemplifies the growing regulatory focus on responsible AI practices, emphasizing data transparency, lineage documentation and robust governance frameworks. To comply with these requirements and stay ahead of global legislation, enterprises should:
- Integrate observability into AI workflows to enhance transparency.
- Build adaptable data governance frameworks to accommodate emerging regulations.
- Collaborate with legal and compliance teams to anticipate regulatory changes and proactively implement necessary adjustments.
By embedding observability and governance into their AI stacks, businesses can achieve compliance and differentiate themselves by establishing trust and reliability in their AI systems.
Embracing Composable AI Architectures
In a rapidly evolving technological landscape, adaptability is essential. A composable AI architecture offers the flexibility to integrate and disassemble tech components as needed. This approach, championed by the MACH Alliance, emphasizes:
- API-first design for seamless integration and interoperability.
- User-centric flexibility to avoid vendor lock-in and reduce costs.
- Agility to quickly adapt to market changes or technological advancements.
- Interoperability to ensure seamless collaboration between different components of the AI stack.
By adopting a composable AI architecture, enterprises can future-proof their infrastructure, ensuring they remain competitive and innovative in an ever-changing digital landscape.
Platform Engineering as a Catalyst for Responsible AI
Platform engineering empowers organizations to build responsible AI by integrating governance, observability and compliance into the AI/ML lifecycle. By enabling self-service access to AI infrastructure with embedded observability tools, platform engineering ensures that ML engineers and data scientists can monitor model performance, data quality and operational metrics in real-time. This transparency fosters accountability and compliance while promoting a culture of continuous improvement.
Leading the Way in Responsible AI Development
As AI continues to revolutionize industries, enterprises must prioritize responsible AI practices to maintain trust, compliance and competitiveness. Platform engineering provides the scalable, adaptable infrastructure needed to achieve these goals, enabling organizations to innovate confidently and responsibly.
By embracing advanced observability solutions, proactive compliance strategies and composable AI architectures, businesses can lead the way in building ethical, transparent and efficient AI systems.
KubeCon + CloudNativeCon EU 2025 is taking place in London from April 1-4. Register now.