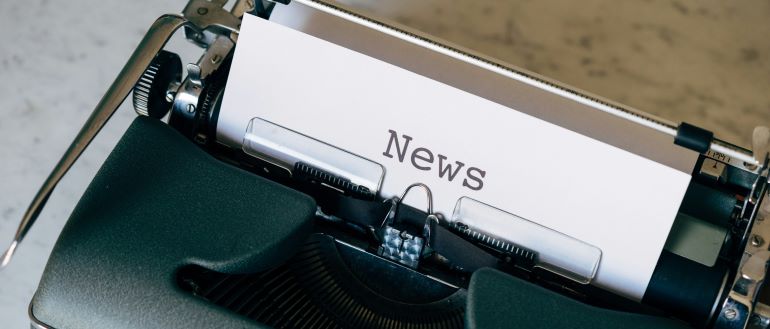
Cribl has unfurled a data lake specifically designed for storing and automatically normalizing telemetry data in a way that reduces total costs by streamlining workflows.
Ed Bailey, principal technical evangelist for Cribl, said the Cribl Lake cloud service provides a unified platform for storing telemetry data that is typically collected by IT operations, DevOps and cybersecurity teams without the need for additional data engineering expertise.
The overall goal is to make it simpler to aggregate that data in a way that, for example, makes it simpler for platform engineering teams to streamline the management of telemetry data on behalf of those teams versus each one setting up their own data lake, he added.
Available on either the Amazon Web Services (AWS) or Microsoft Azure cloud, Cribl Lake is designed to enable teams to query highly distributed telemetry datasets in real time without having to create schemas as it is collected across multiple regions.
In comparison, existing data lakes that many organizations have deployed are designed for more structured data, requiring them to define schemas, deeply understand SQL, and build parsers just to make telemetry data usable, noted Bailey. The Cribl Lake is designed for telemetry data so it eliminates the need to build the complex extract transform and load (ETL) pipelines that data engineers would otherwise be needed to construct across, for example, time series databases and cloud data warehouses, he added.
Organizations that collect telemetry data are being overwhelmed by the sheer volume, resulting in increased costs as they find themselves storing massive amounts that may often never be needed, noted Bailey.
Crib Lake addresses that issue by enabling teams to define storage tiers based on access frequency and retention needs, ensuring real-time access to high-value telemetry data without performance degradation or long retrieval times. It then routes and stores data in the optimal format to reduce costs by 50% compared to other platforms in a way that ultimately provides a foundation for a system of analysis, said Bailey.
It’s not clear at what rate platform engineering teams are unifying the management of telemetry data but as they move to streamline DevSecOps workflows it’s all but inevitable. If organizations hope to enable application developers and cybersecurity teams to proactively address security issues, they need to be able to view the same telemetry data at the same time. Today those teams have separate dashboards providing views in isolated data lakes that make it difficult for the teams to meaningfully collaborate.
More troubling still, the volume of applications generating telemetry data is about to exponentially increase as artificial intelligence (AI) tools increase the number of applications that organizations can build and deploy at scale.
Hopefully, there will also come a day soon when AI also makes it simpler to surface actionable insights from all the telemetry data being collected. The challenge and the opportunity in the short term, however, is finding the most efficient way to expose telemetry data to all the AI models that might need to analyze it.